In the era of Industry 4.0, businesses are increasingly leveraging cutting-edge technologies to streamline their operations. Among these innovations, digital twin technology has emerged as a powerful tool in process automation. It is a virtual replica of a physical system, allowing organizations to simulate, analyze, and optimize performance in real time.
This concept is being applied in various industries, from manufacturing to logistics, significantly enhancing efficiency and reducing operational risks. Interestingly, digital twins have found applications in gaming as well, where real-time simulations enhance user experiences.
For instance, in slots and other online games, the technology enables advanced game mechanics and predictive algorithms. To explore such implementations, click here. However, in industrial and automation, digital twins are changing how companies approach maintenance, process optimization, and predictive analytics.
What Is a Digital Twin?
A digital twin is a digital representation of a real-world entity or process that can be used for monitoring, simulation, and optimization. A high-fidelity model of a physical asset helps companies collect real-time data through IoT sensors, predict failures, improve operational efficiency, and reduce downtime.
This technology is particularly valuable in industries that rely on complex machinery and continuous production processes, such as manufacturing, energy, and logistics. They operate on three core components:
- Physical asset: The real-world object or system being replicated.
- Digital model: A virtual representation that updates based on real-time data.
- Data connectivity: The communication link that enables the digital twin to reflect the live conditions of its physical counterpart.
Implementation in Process Automation
Twins help organizations achieve a high level of operational efficiency by offering detailed insights into process performance. Through simulations, companies can identify bottlenecks and test potential improvements before implementing changes in the physical system. This ability to experiment in a risk-free environment leads to better decision-making and cost savings.
For example, in manufacturing plants, digital twins can replicate production lines and optimize workflows. The system can recommend adjustments that improve efficiency and reduce waste. Similarly, in logistics, they assist in optimizing supply chain processes by predicting potential disruptions and suggesting alternative routes.
Predictive Maintenance
One of the most valuable applications of digital twin technology is predictive maintenance. Traditional maintenance approaches often involve routine inspections or reactive repairs, which can be costly and inefficient. Digital twins, however, enable condition-based monitoring, where maintenance actions are triggered based on real-time performance data.
They can predict equipment failures before they occur. This reduces downtime, extends the lifespan of machinery, and minimizes maintenance costs. In industries like aviation and energy, where equipment failures can have severe consequences, predictive maintenance powered by twins is a game-changer.
Quality Control
Digital twins contribute significantly to quality assurance processes.
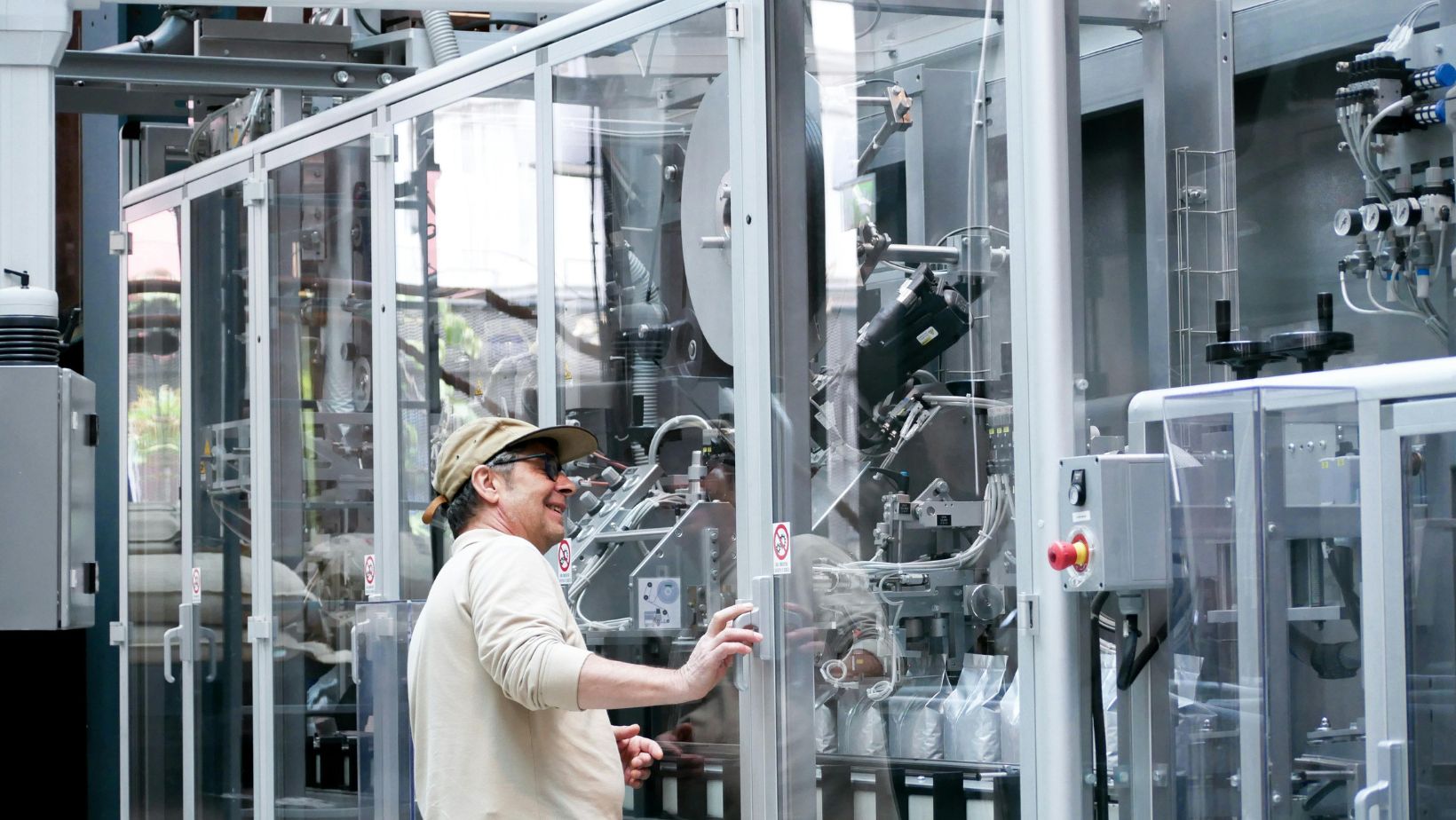
Replicating production conditions and analyzing deviations help identify defects and address quality issues before products reach the market. In the pharmaceutical industry, for example, they help ensure compliance with strict regulatory standards by monitoring drug production processes in real time.
Decision-Making with Data-Driven Insights
The ability to collect and analyze vast amounts of data is another major advantage of digital twins. Through machine learning algorithms, they can uncover patterns and provide actionable insights. This data-driven approach enhances strategic decision-making and allows companies to adapt to changing conditions more effectively. For instance, in smart cities, twins are used to model urban infrastructure.
Challenges in Adopting Digital Twin Technology
Despite its advantages, implementing digital twins comes with certain challenges:
- High initial investment: Developing a comprehensive twin system requires significant investment in IoT sensors, cloud computing, and AI-driven analytics.
- Data integration complexities: Aggregating and synchronizing data from multiple sources can be challenging, particularly in legacy systems.
- Cybersecurity risks: As digital twins rely on constant data exchange, they can become targets for cyber threats. Ensuring robust security measures is critical to protect sensitive information.
- Skill gaps: Organizations may struggle to find professionals with expertise in digital twin technology, requiring investment in workforce training.
To Sum Up
The future of digital twin technology looks promising, with advancements in AI, edge computing, and 5G connectivity set to enhance its capabilities further. They will play an increasingly vital role in driving efficiency and innovation. While challenges remain, continuous technological advancements are making digital twins more accessible and powerful.